Disentangling impact of capacity, objective, batchsize, estimators, and step-size on flow VI
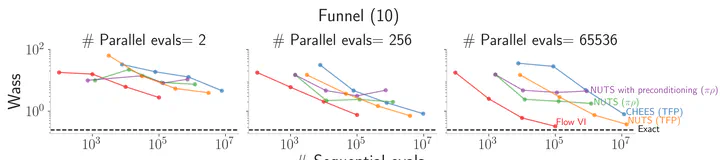
Abstract
Normalizing flow-based variational inference (flow VI) is a promising approximate inference approach, but its performance remains inconsistent across studies. Numerous algorithmic choices influence flow VI’s performance. We conduct a step-by-step analysis to disentangle the impact of some of the key factors: capacity, objectives, gradient estimators, number of gradient estimates (batchsize), and step-sizes. Each step examines one factor while neutralizing others using insights from the previous steps and/or using extensive parallel computation. To facilitate high-fidelity evaluation, we curate a benchmark of synthetic targets that represent common posterior pathologies and allow for exact sampling. We provide specific recommendations for different factors and propose a flow VI recipe that matches or surpasses leading turnkey Hamiltonian Monte Carlo (HMC) methods.
Type
Publication
Arxiv