Abhinav Agrawal
Building AI products | GenAI expert | Probablistic ML PhD
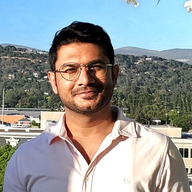
Hi there! I recently defended my PhD and am officially in the industry job market. I bring a unique blend of deep technical expertise, zero-to-one product experience, and excellent communication skills. Read more below or set up a short call :)
I recently completed my PhD in computer science at the University of Massachusetts Amherst, advised by Prof. Justin Domke. My work leveraged generative modeling approaches for designing efficient sampling methods.
I remain broadly interested in artificial intelligence. Recently, I led product at SigIQ.ai to build India’s leading AI tutor app, where I managed a team of designers, engineers, and AI experts. Prior to that, I spent a wonderful summer designing generative-model-based causal models for the Causality and Machine Learning team at Microsoft Research Redmond. I spent another summer as an applied scientist intern working on deep-learning-based ranking models for the personalization team at Amazon.
Before my graduate studies, I spent four amazing years at IIT Kanpur acquiring a background in computer vision, deep learning, and generative models. During this time, I was mentored by Prof. Piyush Rai, Prof. Tanay Guha, and Prof. Vinay P. Namboodiri (undergraduate thesis advisor).
Aside from work, I enjoy keeping myself fit, reading books, making people laugh, and playing board games. I cherish deep, philosophical discussions, including but not limited to AI and its implications :) Feel free to contact me, or set up a quick call.
Selected Publications
- Preprint
- NeurIPS ’21